DC-11: Julien Amblard
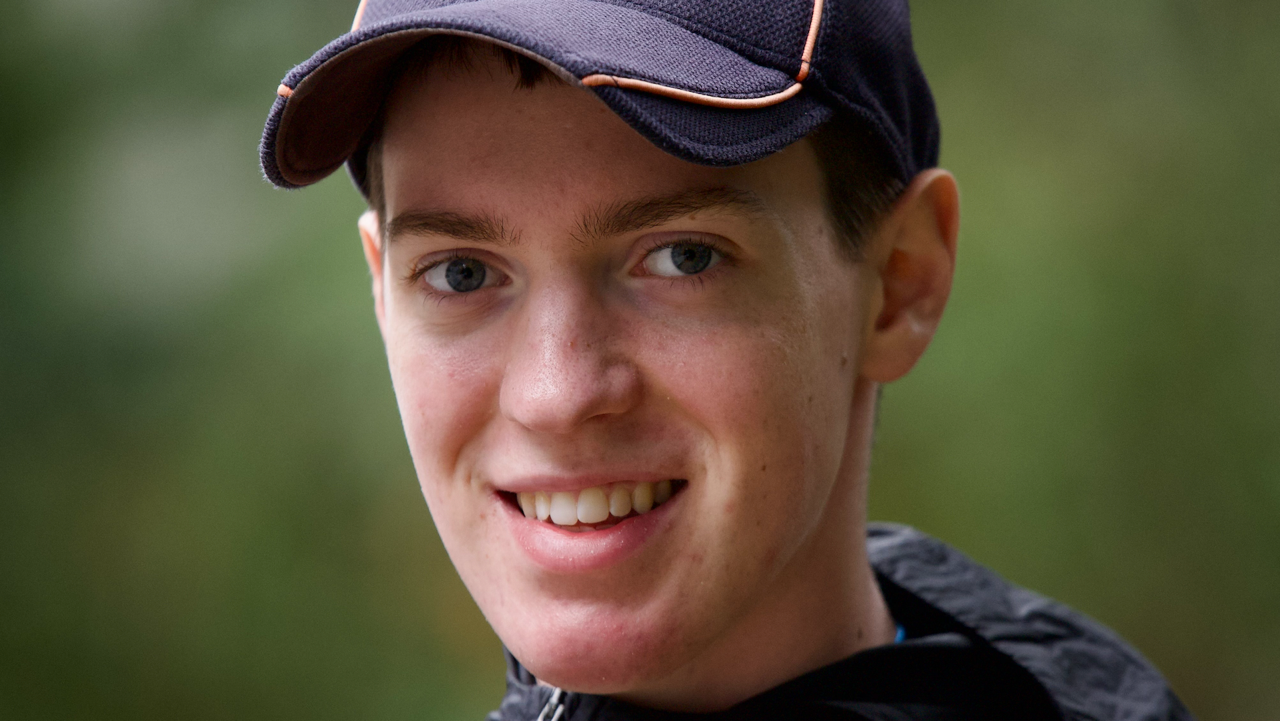
Contact
E-mail: julien.amblard16@imperial.ac.uk
Project
Symbolic machine learning solutions for abnormal event identification
Host organization
IMPERIAL
Supervisors
Prof. Alessandra Russo (Main, IMPERIAL); Prof. Gürkan Sin (co-supervisor, DTU)
Duration
36 months
Objectives
The main objective is to develop advanced neuro-symbolic ML methods to learn interpretable models of causality of abnormal events through novel ways of representing and incorporating domain knowledge (e.g., process flow diagram, knowledge representation of the chemical process) with historical data about hazard and operability studies. These models aim to define deviations from normal operations.
The use of domain knowledge guarantees consistency of the learned models with underlying chemical and system processes, and the ability to learn from a limited amount of hazard data. The learned model will be used to establish a platform for an online early warning system capable of avoiding abnormal situations and better controlling the system dynamics. The research on this novel interpretable ML method will take place at IMPERIAL, and the platform will be implemented on large datasets of historical data from hydrogen production and the liquefaction process during industrial secondment (KAIROS).
Expected results
- The development of a new formalism for representing domain knowledge about dynamic systems together with qualitative notions of abnormalities. This formalism will underpin the reasoning and learning about the causes and consequences of events in terms of dynamic changes over continuous and discrete variables,
- The development of a neuro-symbolic ML learning algorithm that exploits knowledge about chemical processes and process flow diagrams represented using the newly developed formalism, to learn from historical data of past hazards, models of causes, and consequences of abnormal events identification,
- Application of platform modeling software to renewable hydrogen process (control room alarm tracking) with adequate counter-action planning.
Planned secondments
- KAIROS, M17, 3 months: Data collection and process/plant understanding of alarms, abnormal events, and safety-related issues
- DTU, M25, 2 months: Training on model-based and hybrid approaches for data analysis for process safety