DC-6: Robson Wilson Silva Pessoa
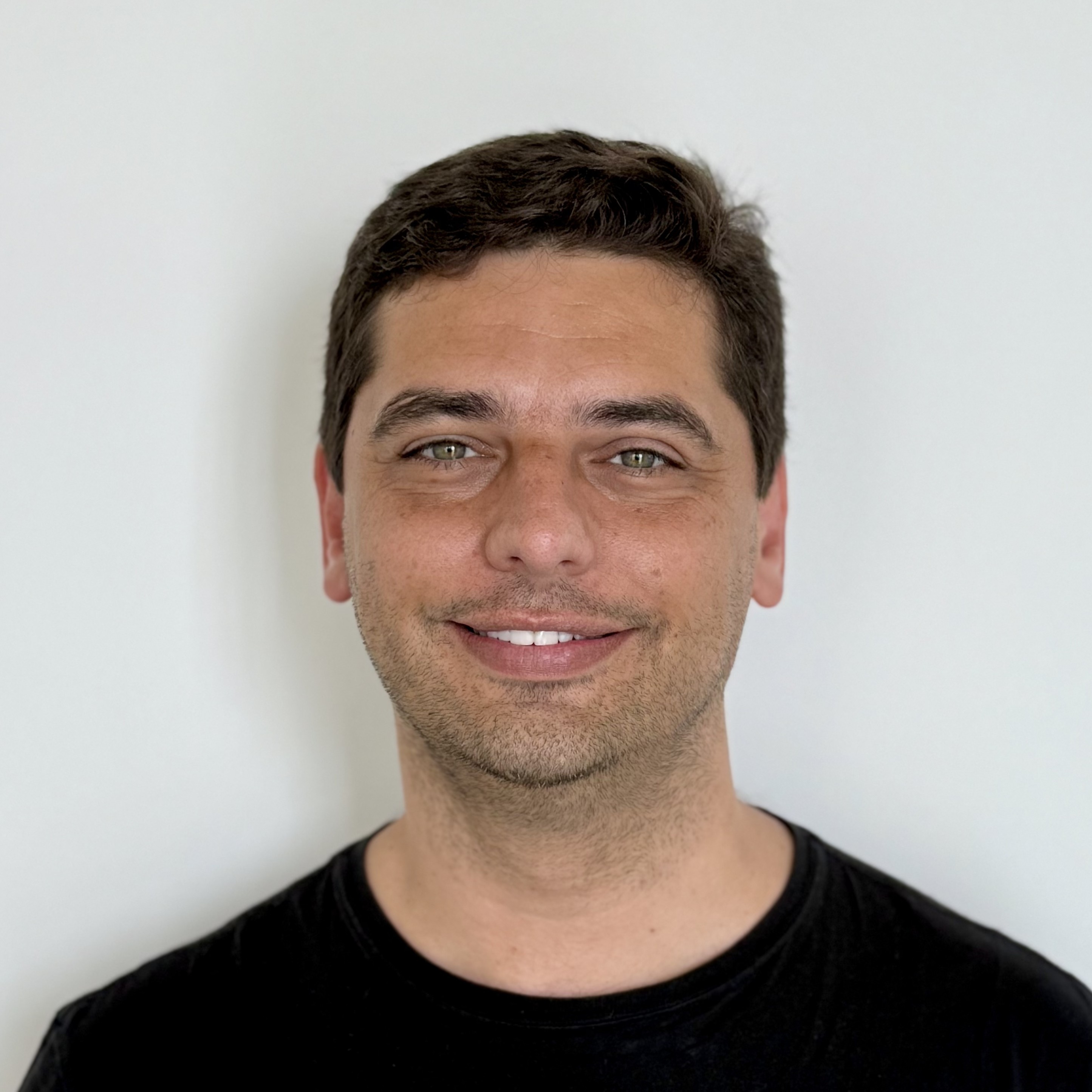
Contact
E-mail: robson.w.s.pessoa@ntnu.no
Project
Hybrid, regressible, and robust machine learning solutions for abnormal event identification
Host organization
NTNU
Supervisors
Prof. Johannes Jäschke & Assoc. Prof. Idelfonso Nogueira (Main, NTNU); Prof. Gürkan Sin (co-supervisor, DTU)
Duration
36 months
Objectives
The primary goal entails the formulation of a hybrid, regressible, and robust Machine Learning (ML) methodology designed to recognize irregular events by assimilating phenomenological understanding of the process, historical hazard, and operability data.
The ML models will be crafted following an uncertainty-aware approach to enhance their reliability. This will involve identifying epistemic and aleatory uncertainties during the modeling phase and subsequently incorporating these uncertainties into the model predictions. The main purpose of these models is to identify deviations accurately and reliably from standard operations, thereby aiding decision-making processes. These models will also accommodate online learning based on continually generated data. This online learning will enhance the model's reliability by monitoring the models' predictions vis-à-vis measured data and updating the models when required.
The security of the online learning process and system integrity will be maintained through multiple digital replications of the model's probability distribution. This novel research will be applied to extensive historical datasets from the LNG regasification process during the industrial secondment at KAIROS.
Expected results
- Curate data, categorize historical data's abnormal events and evaluate uncertainties,
- Identify hybrid ML models and characterize epistemic uncertainty,
- Determine a probability distribution of ML models, considering both epistemic and aleatory uncertainties.
- Employ multivariate statistics and sparse regression tools to extract interpretable mathematical functions from ML models,
- Test and validate the online learning capabilities of the models against a dataset not employed in the identification process,
- Implement platform modeling software for tracking control room alarms, complemented with appropriate counteraction planning.
Planned secondments
- KAIROS, M17, 3 months: Data collection and process/plant understanding of alarms, abnormal events, and safety-related issues
- DTU, M25, 2 months: Training on model-based and hybrid approaches for data analysis for process safety