DC-7: Mohammadreza Jahanbakhsh
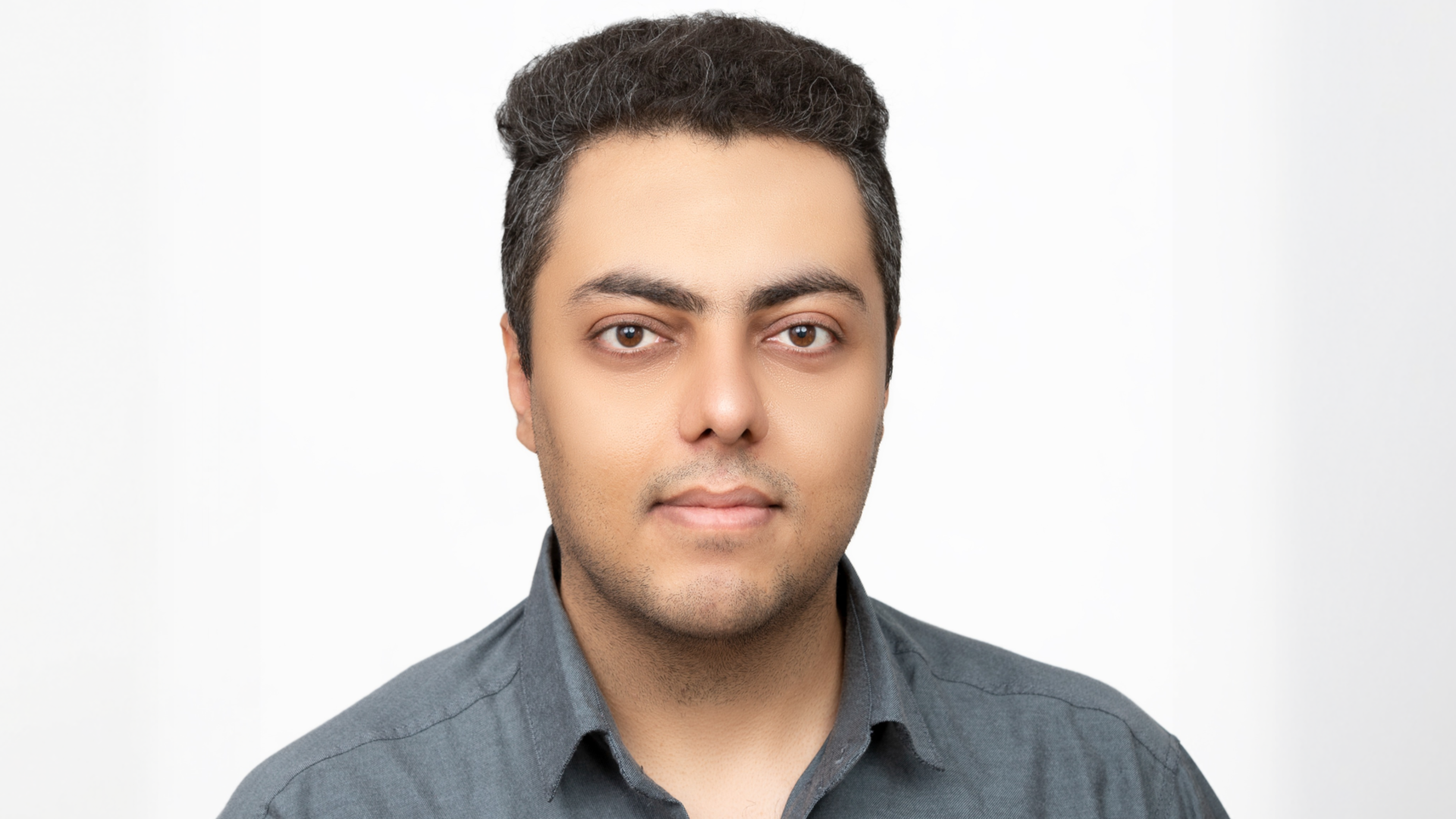
Contact
E-mail: mjahanbakhsh24@ku.edu.tr
💼LinkedIn 🆔ORCID 🏛️ResearchGate 📖Google Scholar
Project
Physics-aware machine learning for control of chemical processes
Host Organization
KU
Supervisors
Prof. Can Erkey (Main, KU); Prof. Gürkan Sin (co-supervisor, DTU)
Objectives
- Embed physics into neural network training for improved reliability.
- Develop custom ML architectures (e.g., RNN, LSTM) for large time series data.
- Create a framework to build, update, and validate predictive control models.
- Explore reinforcement learning for optimal safety and economic trade-offs.
Project Description
This project aims to revolutionize control strategies in the chemical processing industry by integrating physics-informed insights into state-of-the-art machine learning models. The central idea is to incorporate fundamental process physics directly into the training phases—both offline and online—of neural network architectures such as recurrent neural networks (RNN) and long short-term memory (LSTM) networks. By doing so, the project seeks to enhance predictive performance and reduce the risk of suboptimal training, resulting in more robust and reliable control models.
A key component of the project is the development of a systematic framework that leverages extensive time series data from production plants. This framework will be used to build, update, and validate predictive analytics algorithms that are subsequently embedded into model-based closed-loop control systems. These systems include classical, nonlinear, and economic model predictive control formulations tailored to specific industrial challenges. Moreover, the project will explore extending these methods to reinforcement learning, enabling the algorithms to autonomously learn the best mitigation actions that optimize both safety and economic outcomes.
The proposed methodologies will be rigorously tested against conventional control structures in a case study focusing on the urea and hydrogen processing sectors. Through planned secondments at DTU and NOVOTEC—providing advanced training in uncertainty analysis and LNG case study applications—the project also emphasizes knowledge transfer and practical implementation. Ultimately, by marrying fundamental physics with advanced machine learning techniques, the project aspires to deliver significant improvements in process control, ensuring operational efficiency, safety, and economic viability for industrial plants.
Relevant Background
- Chemical Engineering Bachelor Degree
- Chemical Enginering master degree in Shiraz University
- Masetr thesis subject: CO2 decomposition in DBD plasma Rector
- Proficient in a wide range of modeling and simulation tools, including Python, MATLAB, Aspen Plus, COMSOL Multiphysics, ANSYS Fluent and Implementation of machine learning models in chemical engineering problems
Publications
- Polyurethane foam: A novel support for metal oxide packing used in the non-thermal plasma decomposition of CO2, H Taghvaei, E Pirzadeh, M Jahanbakhsh, O Khalifeh, MR Rahimpour Journal of CO2 Utilization 44, 101398
-
A Cutting-Edge Approach: Leveraging Deep Sequence Learning for Simultaneous CO2 Capture and In-Situ Utilization in a Highly Efficient Syngas and Ultra-Pure Hydrogen Generation Process M.Piroozmand, M. Jahanbakhsh, A. Hafizi SSRN.