DC-12: Christos Ziakas
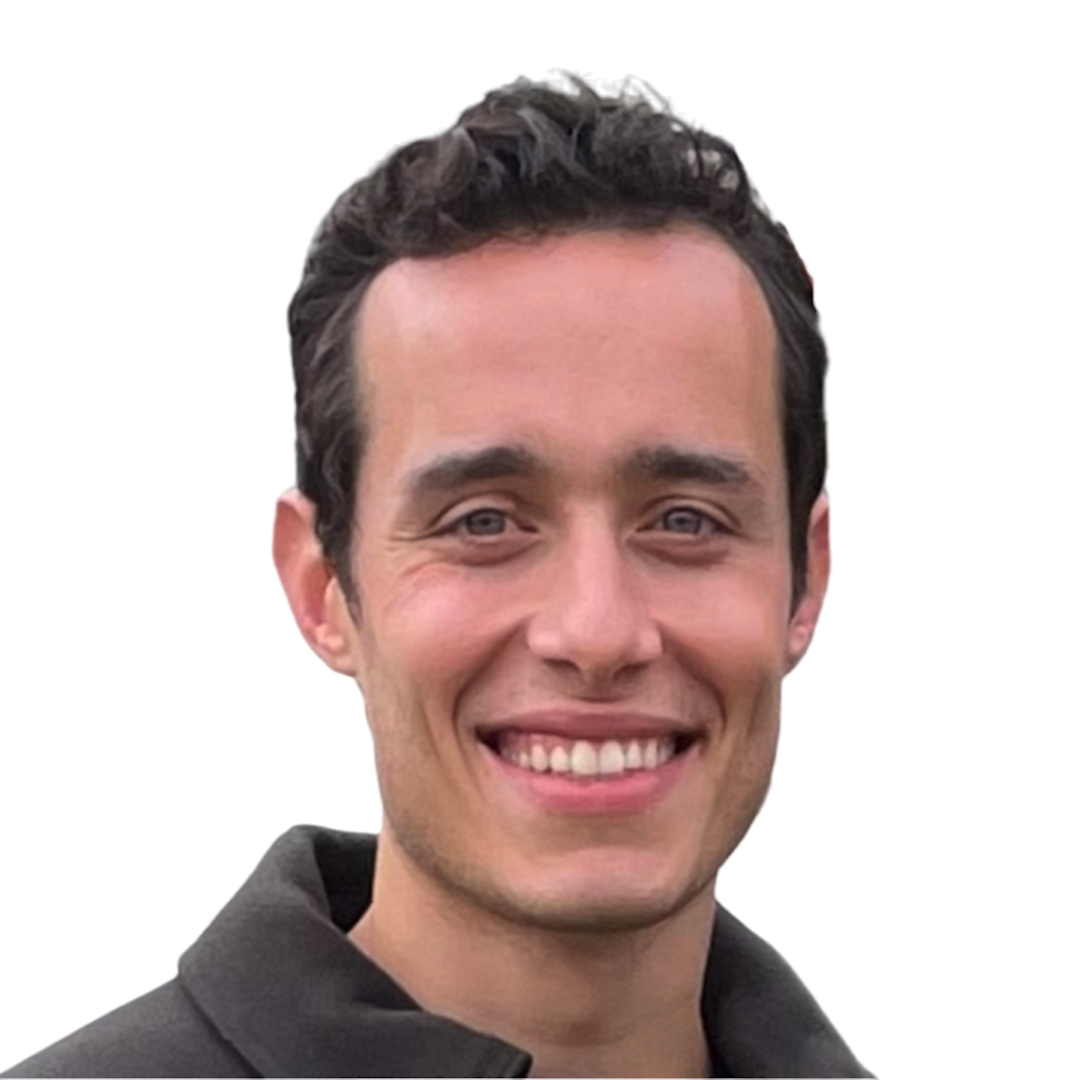
Contact
E-mail: c.ziakas24@imperial.ac.uk
Project
Closed loop integration of hybrid machine learning and automated adaptation of chemical processes for safe process operation
Host organization
IMPERIAL
Supervisors
Prof. Alessandra Russo (Main, IMPERIAL); Prof. Eulàlia Planas (co-supervisor, UPC)
Duration
36 months
Objectives
The overall objective is to develop a closed-loop integration of the monitoring, diagnosis, and mitigation of abnormal events thus contributing to safe plant operation and better control of the system. Neuro-symbolic online ML algorithms will be developed that use online monitoring data to learn models for predicting the most likely diagnosis of abnormal events and the best mitigation of their causes. A neuro-symbolic inference algorithm will be used to combine monitoring data with learned models about abnormal events to predict the causes and consequences of potential deviations.
These predictions can be used by a neuro-symbolic RL algorithm to determine the best course of action to perform to mitigate the causes of the predicted deviation and support model-based process adaptation to minimize the occurrence of abnormal events, thus leading to improved process safety. The key challenge is determining mitigation mechanisms that are consistent with the underlying process-related domain knowledge.
The results will be applied to a case study from the urea processing industry in collaboration with NOVOTEC.
Expected results
- Novel neuro-symbolic inference algorithm capable of reasoning about causal and consequences models of abnormalities, expressed over continuous and discrete variables, to perform diagnosis of abnormalities based on online monitoring data,
- Novel neuro-symbolic reinforcement learning algorithm capable of learning best mitigation actions from online data using models of causes and consequences of abnormalities,
- An integrated “monitoring-learning-acting” platform that integrates real-data analytics tools with neuro-symbolic inference and learning of model-based process adaptation,
- Application to a case study to evaluate the efficacy of the adaptation in terms of safety risk minimization, conservative changes, and undesired impacts.
Planned secondments
- UPC, M13, 2 months: Training on QRA modeling principles and QRA software application possibilities
- NOVOTEC, M30, 3 months: Training on design of web-based specifications for software services