DC-4: Jingkang Liang
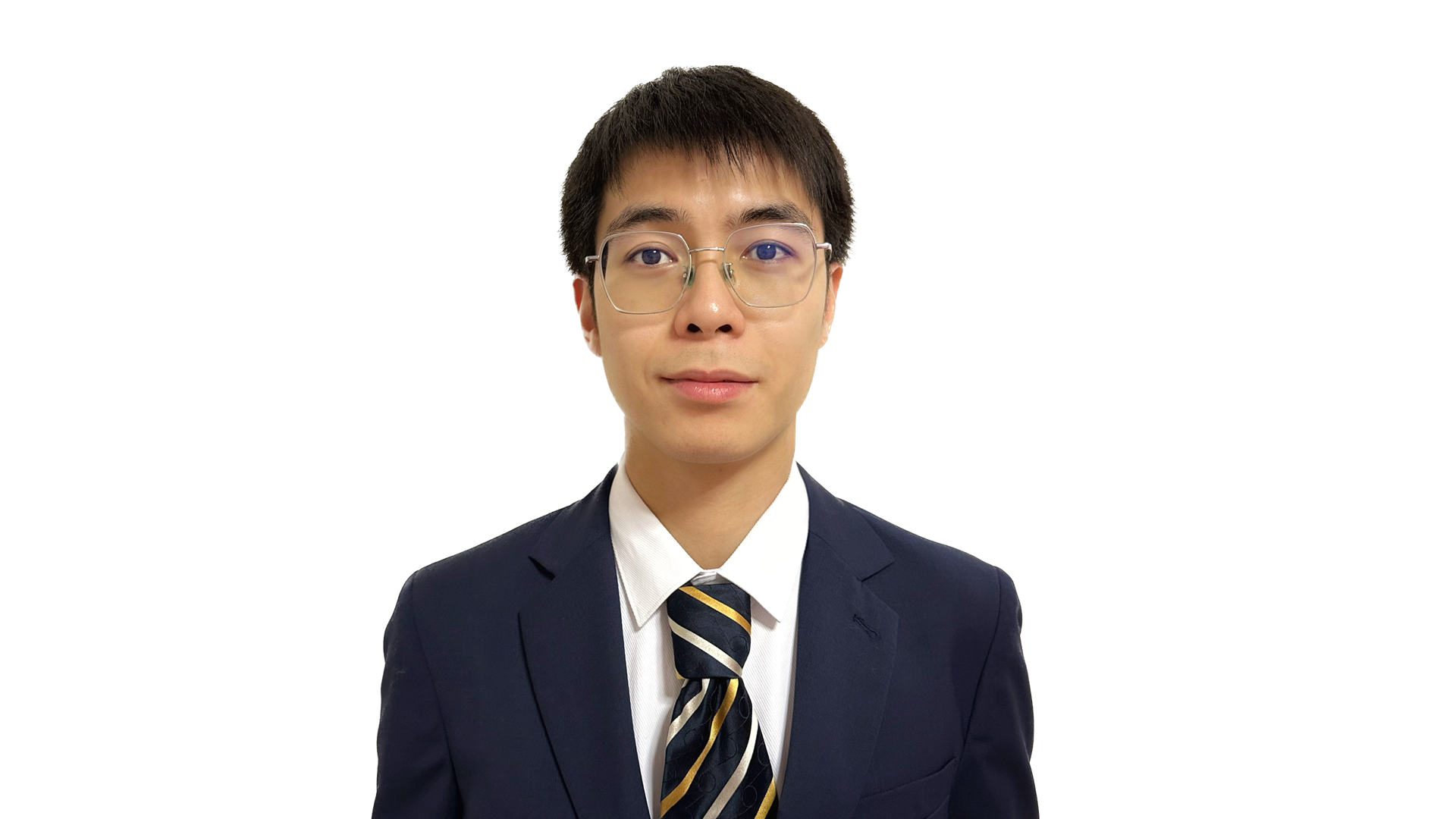
Contact
E-mail: jinlia@kt.dtu.dk
LinkedIn
Project
Statistical machine learning and big data analytics for online risk monitoring and fault diagnosis
Host organization
DTU
Supervisors
Prof. Gürkan Sin (Main, DTU); Prof. V. Venkatasubramanian (co-supervisor, COL); Dr. Claus Myllerup (co-supervisor, KAIROS)
Duration
36 months
Objectives
The overall objective is to investigate how quantitative methods can be utilized in a qualitative framework to provide online Quantitative Risk Assessments to operators for decision-making support. Furthermore, aiming to investigate how qualitative and quantitative evidence can be produced online from sensor signals in combination with a QRA, to guide operators’ decision-making in abnormal situations.
The DC will work on applying the results to a hydrogen liquefaction and processing facility as the industrial use case.
Expected results
-
Online QRA for an operational plant or historical data from the very same plant,
-
Identification and application of suitable quantitative methods for probabilistic estimation of individual risk per annum,
-
Benchmarking of method(s) suitable for producing qualitative and quantitative evidence to reduce situational uncertainty about risk and severity,
-
Development of a method for visualizing QRA results online for operators to aid with decision support.
Planned secondments
- KAIROS, M13, 3 months: Training on fault diagnosis and monitoring for process safety applications
- Risktec, M28, 2 months: Big data analytics validation on renewable hydrogen case study