DC-2: Niklas Groll
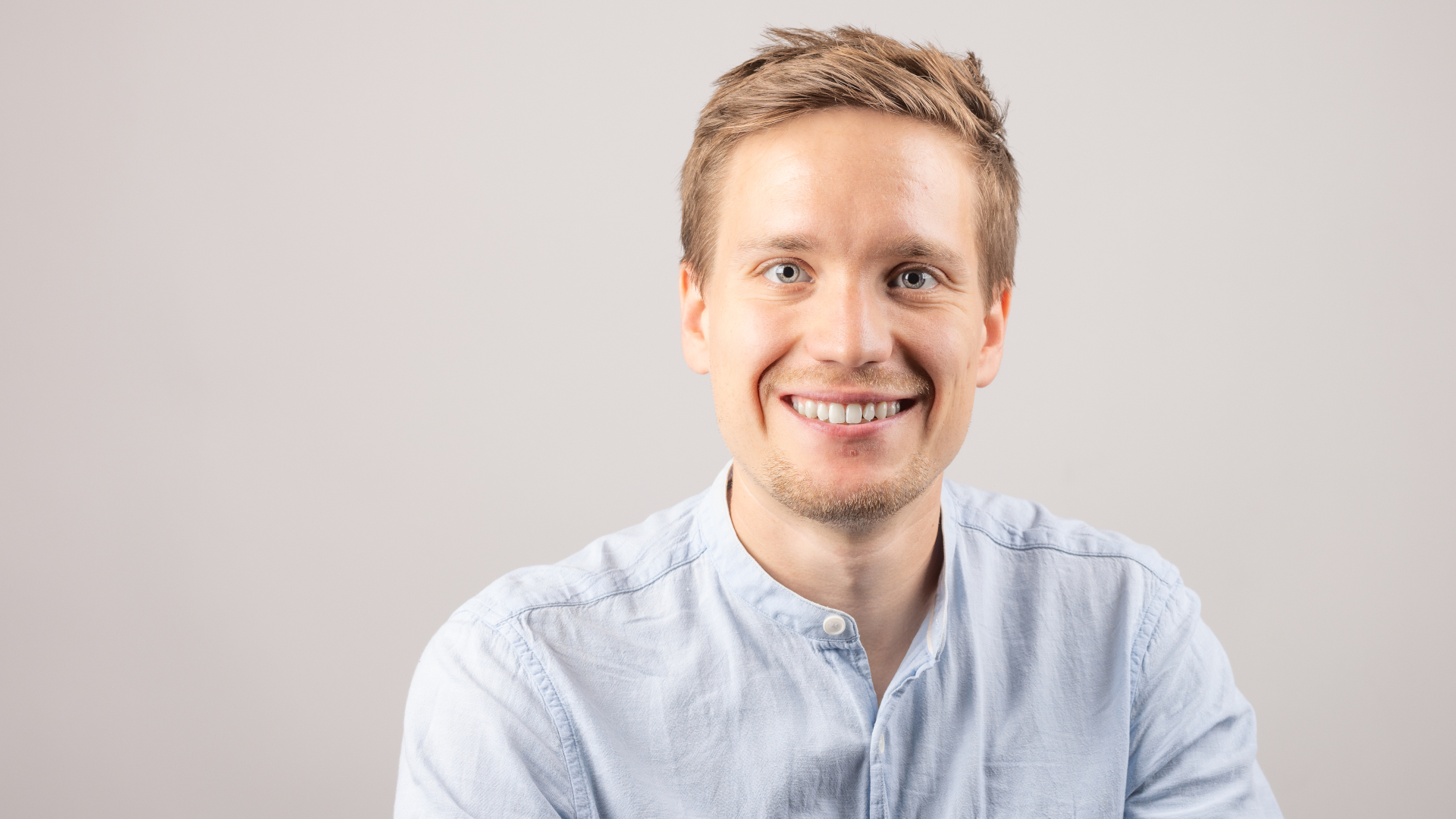
Contact
E-mail: nigro@kt.dtu.dk
💼LinkedIn 🆔ORCID 🏛️ResearchGate 📖Google Scholar
Project
Hybrid approaches for smart integration of AI/deep learning with model-based algorithms for online risk monitoring
Host Organization
DTU
Supervisors
Prof. Gürkan Sin (Main, DTU); Prof. Alessandra Russo (co-supervisor, IMPERIAL)
Objectives
- Design of a novel simulation benchmark model based on green ammonia production
- Assess process control and safety methodologies with the help of the novel ammonia benchmark simulation model
- Monitor potential risks and unsafe states in green ammonia production by hybrid modeling techniques
- Apply innovative risk monitoring methodologies to industrial case studies
Project Description
My project (ProSafe DC‐2) targets the application of hybrid modeling, combining the advantages of first‐principal models with those of data‐driven models for risk monitoring. Pure knowledge‐based models often lack preciseness for real processes and tend to become very nonconvex and difficult to solve due to increasing process complexity. While pure data‐driven algorithms usually feature fast calculations, they underperform for states with poor data availability. In contrast, hybrid models promise fast and accurate predictions, which are essential for process control and monitoring, and feature the possibility of extrapolating former unrecorded states, e.g., in cases of dangerous process conditions due to failures.
Our research project breaks down into three milestones: First, the requirement of an updated simulation benchmark model, encompassing typical features of emerging processes, e.g., sustainable feedstocks and renewable energy consumption, is essential. The simulation benchmark model will serve as a platform to develop and test novel process safety methodologies later. As use cases, processes part of the rising renewable hydrogen economy are particularly suitable because of their impact on the green process transition and their high complexity because of the combinations of chemical and electrochemical reactions and the dynamic process behavior based on renewable energy availability. In the second step, we develop novel hybrid methodologies connecting mechanistic models based on knowledge and machine learning models based on process data for the application of risk monitoring. The performance of the novel hybrid models can be compared to traditional process monitoring techniques and pure data‐driven models utilizing the former established benchmark simulation model. For the last milestone, the operational range of the developed hybrid methodology for risk monitoring should be extended to be applicable for industrial use cases featuring real process data.
Overall, my project aims to significantly contribute to safer process operations of innovative processes by providing fast and precise risk monitoring technology based on hybrid modeling techniques. Our work will close the gap between traditional modeling based on knowledge and emerging data‐driven algorithms in close collaboration with other doctoral candidates and partners from the ProSafe initiative.
Relevant Background
- BSc Mechanical Engineering (2017-2022) and MSc Chemical Engineering (2022-2024) from RWTH Aachen University
- Exchange studies at Lund University (Sweden), ETH Zürich (Switzerland), and NTNU Trondheim (Norway)
- Master’s thesis: Dynamic Scheduling with Tracking Nonlinear Model Predictive Control for Flexible Biodiesel Production
- Student Research Assistant in the fields of Life Cycle Assessment, Sector Coupling in Sustainable Energy Systems, and Power-to-X (2019-2023)
Publications
- Voelker, S., Groll, N., Bachmann, M. et al. Towards carbon-neutral and clean propulsion in heavy-duty transportation with hydroformylated Fischer–Tropsch fuels. Nat Energy 9, 1220–1229 (2024). https://doi.org/10.1038/s41560-024-01581-z